Introduction
The assessment of peripheral lymphocyte subpopulations is one of the basic immunological tests performed in medical diagnostic laboratories. Thanks to the assessment of surface antigens, it allows for the division of lymphocytes into individual subpopulations, such as T lymphocytes (including helper and cytotoxic T lymphocytes), B lymphocytes, natural killer (NK) cells and NKT lymphocytes. It is initially performed, of course, with all suspicions of immunodeficiency, but it is also used in many other fields of medicine.
The use of immunophenotyping is crucial for diagnosis of leukaemias and lymphomas. Immunophenotype is one of the factors used in international classification of leukaemias, because it allows one to accurately differentiate cells and distinguish the lineage attribution of the disease [1, 2]. It could be successfully used to monitor the response to leukaemia treatment as well [3]. Other than that, the use of flow cytometry was proven crucial for assessing the prognosis and lineage origin of myelodysplastic syndromes (MDS) [4].
The assessment of lymphocyte subpopulations, especially Th lymphocytes (CD4+), is included in the recommendations for human immunodeficiency virus diagnosis and evaluation of the treatment’s effectiveness. The progress of the infection is tracked by the depletion of CD4+ lymphocytes [5]. On the other hand, the assessment of NK cells may be used to evaluate non-haematological tumours considering factors such as treatment effectiveness [6]. This subpopulation is also crucial in the response to pathogens; thus the assessment of its function is a helpful diagnostic tool [7, 8]. Additionally, studies indicate the potentially disruptive use for immunophenotyping in the diagnosis of rare inflammatory and autoimmune diseases, especially those that do not fit into standard diagnostic procedures [9].
Another field that widely uses the flow cytometry methods is allergology. The cells mostly responsible for the immediate allergic response are basophils; thus the basophil activation test (BAT), with the use of flow cytometry, is commonly performed during allergy diagnosis [10]. Since lymphocytes are also involved in hypersensitivity reactions, the evaluation of certain subpopulations is included in their differentiation and diagnosis [11].
Due to the fact that it is a common test and therefore performed in many laboratories on different analysers and by different diagnosticians, it is important that the results obtained in different places or with the use of different analysers and software do not differ significantly from each other and the testing technique does not affect the diagnosis and the course of treatment of the patients.
The aim of the study was to compare the results of the assessment of peripheral blood lymphocyte subpopulations performed by different diagnosticians on two flow cytometers – BD FACSCanto II (Becton Dickinson) and BD FACSLyric (Becton Dickinson) – and assess whether the differences could influence the clinical judgement and procedures.
Material and methods
The study included 93 paediatric patients hospitalised in the Children’s Teaching Hospital of the Medical University of Warsaw, in whom physicians ordered an assessment of the subpopulation of peripheral blood lymphocytes as a part of routine hospital diagnostics. The test was performed by a laboratory worker on one analyser and then independently repeated by another researcher on a second analyser. The study also included the evaluation of 9 control samples (multi-check control), which is performed in the laboratory once a week. Altogether 102 samples were evaluated. All of them were analysed in percentages and for 58 of them also absolute counts (using Tru-count tubes) were analysed.
All necessary controls of the analysers’ operation, such as cytometer set-up, tracking beads (CST) and settings update, were performed in accordance with the recommendations of the quality control. CST was performed every 24 hours and every two months we performed a setting update.
The samples were prepared by mixing 50 µl of K3EDTA whole blood with 10 µl of labelled antibody mixture (Multitest 6-color TBNK kit: CD3 FITC / CD16 PE + CD56 PE / CD45 PerCP-Cy5.5 / CD4 PE-Cy7 / CD19 APC / CD8 APC-Cy7, Becton Dickinson) and left for 20 minutes of incubation at room temperature without access to light. Then, 1000 µl of lysing solution (EasyLyse, DAKO) was added and left for another incubation for 10 minutes at 4-8°C. Immediately after the incubation the samples were ready for measurement.
Samples were analysed on the BD FACSCanto II, with FACS Diva 2.0 software and BD FACSLyric, with FACSSuite software, both Becton Dickinson.
An example of the results obtained with both analysers and the methods of gating the population is shown in Figure 1.
The statistical analysis was conducted using Microsoft Office Excel and StatSoft Statistica 13 software. To analyse the agreement between the two assays we used the Bland-Altman plot. We presented the distributions of differences between the two compared methods using a mountain plot. Spearman’s rank correlation examined the strength and direction of monotonic association between two variables. We performed a Passing-Bablok regression test for nonparametric regression analysis.
Results
In this study we evaluated the differences between lymphocyte subpopulation percentage and absolute values obtained from two flow cytometry analysers. We tested the strength of the association between our two variables using Spearman’s rank correlation. The correlation coefficients for T, Tc, Th, B and NK cells showed a strong positive correlation (> 0.96) between analysers’ results for both absolute and percentage values. For NKT cells, the correlation coefficients were lower, 0.856290 for percentage values and 0.807840 for absolute values, suggesting a weaker correlation between these variables (Table 1). All the calculations were performed with a level of significance p < 0.05.
Table 1
Spearman’s rank correlation coefficient values for percentage and absolute values of all examined lymphocyte subpopulations. All of the coefficient values indicate a strong positive correlation between both analysers
Bland-Altman plots were used to analyse the agreement between our two assays. They showed that for T, Tc and Th cells, for both percentage and absolute values, as well as B cell absolute values, the results obtained from FACSLyric were slightly higher than those from FACSCanto II. However, for NK and NKT, both percentage and absolute values, as well as B cell percentage values, FACSLyric results were slightly lower than FACSCanto II results. Based on the Bland-Altman plots, we calculated the Bland-Altman coefficient and assessed the level of similarity between measurements (Table 2). We considered the results comparable when the coefficient’s value was lower than 5%.
Table 2
Bland-Altman coefficient values for percentage and absolute values of all examined lymphocyte subpopulations. Six values, which constitute 50% of all the parameters, were lower than 5%, indicating a good comparability between results
Lymphocyte subpopulation | Bland-Altman coefficient | |
---|---|---|
Percentage values | Absolute values | |
CD3+ | 4.90% | 5.17% |
CD3+8+ | 2.94%* | 8.62%** |
CD3+4+ | 3.92% | 6.90% |
CD19+ | 7.84% | 6.90% |
CD3−16+56+ | 4.90% | 3.45% |
CD3+16+56+ | 4.90% | 6.90% |
We observed the best comparability in the measurements of Tc lymphocyte (CD3+8+) percentage values. However, the highest Bland-Altman coefficient was noted in the absolute values of the same parameter, indicating the worst comparability between the two analysers (Fig. 2).
Fig. 2
Comparison of Bland-Altman plots for percentage Tc subpopulation value (A) with the lowest Bland-Altman coefficient and absolute Tc subpopulation value (B) with the highest Bland-Altman coefficient
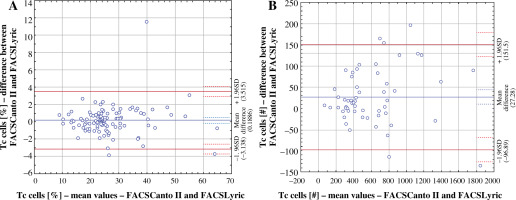
The Passing-Bablok regression analysis showed that all parameters had a slope value very close to 1, meaning there is good comparability between methods (Table 3). The parameter that had the strongest correlation between methods was Tc cell percentage value assessment, and the weakest correlation was discovered considering NKT cell percentage values (Fig. 3).
Fig. 3
Comparison of Passing-Bablok regression plots between Tc cell percentage value with the strongest correlation between the two methods (A) and NKT cell percentage value with the weakest correlation (B)
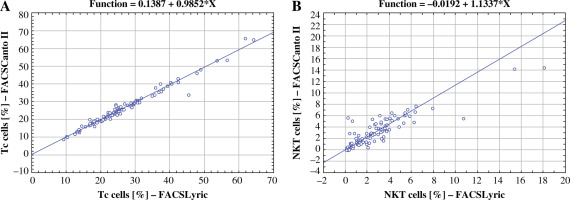
Table 3
Passing-Bablok regression slope values. All of the analysed parameters showed linear dependence and strong correlation with slope values close to 1
Lymphocyte subpopulation | Passing-Bablok regression slope values | |
---|---|---|
Percentage values | Absolute values | |
CD3+ | 1.0334 | 0.9438 |
CD3+8+ | 0.9852 * | 0.9623 |
CD3+4+ | 0,9775 | 0.9402 |
CD19+ | 1.0336 | 0.9728 |
CD3−16+56+ | 1.0576 | 1.0364 |
CD3+16+56+ | 1.1337 ** | 1.0586 |
Additionally, we created mountain plots to illustrate the comparability of the results, as shown in Figure 4.
Discussion
The popularity of immunophenotyping by flow cytometry means that many laboratories and research centres use this technique on a daily basis. This leads to the development of technology and techniques, potentially creating difficulties with the comparability of the results. The extensive use of this method raises a question about the differences between used methods and analysers. In this study, we compared two well-known flow cytometers to examine the occurrence of differences and establish whether they may influence the results and the clinical judgements based on them. The results from both analysers in our study were considered comparable, with only one statistical method showing some deviations.
A comparative study, similar to the one that we conducted, was performed by Xu et al. and showed no statistically significant differences in lymphocyte subpopulation assessment considering three different analysers, FACSCanto II, FACSLyric (the two analysers we compared), and additionally FACSCalibur, as well as different research centres [12]. Comparable results were also obtained in another study regarding immunophenotyping by the same analysers included in our study – FACSCanto II, FACSLyric – both in blood samples from relatively healthy subjects and from ones with immune and haematological disorders [13]. Both of the mentioned studies utilised the two flow cytometry analysers used in this study. In order to eliminate potential differences and mistakes, strict standardised procedures have to be followed for the sake of reliable, comparable and accurate results [14].
Murugesan et al. compared two methods of CD34+ hematopoietic stem cell assessment, using one flow cytometry analyser to conduct both analyses, and found no significant differences [15]. Another study by Avecilla et al. also presented a comparison of CD34+ cells in peripheral blood, although they included an automated haematology analyser in comparison to the reference flow cytometry method. The results showed that the haematological analyser can be successfully used as a substitute for laboratories not equipped with a reference method [16].
A comparison of results for CD4+ count made by Kosasih et al. showed comparable results between two flow cytometry analysers, one of which was used in our study as well. However, the authors pointed out the discrepancy for some of the subjects, a possible cause being the differences in gating techniques between analysers [17]. A similar study was performed by Negedu-Momoh et al., who compared the point-of-care (POC) analyser BD FACS-Presto, used to evaluate the CD4 subpopulation, while monitoring HIV infection with a reference flow cytometry method – BD FACSCount. Their study showed good comparability between analysers, as well as no significant differences between the results of tests run by laboratory specialists and non-laboratory operators [18]. On the other hand, Moran et al. found a statistically significant overestimation of CD4+ count using BD FACSPresto in comparison to BD FACSCount, considering an examination of capillary and venous blood [19]. In contrast to that, the study by Kingwara et al. indicated an underestimation of BD FACSPresto, in the case of assessing CD4+ in venous blood [20]. Another two studies performed by Lu et al. [21] and Makadzange et al. [22] investigated a validation process of CD4+ lymphocyte evaluation using the same analyser as the previous research, BD FACSPresto, and its comparison with BD FACSCalibur. The results of both studies indicated the good consistency between these two analysers. It is in agreement with two separate, analogous studies by Elharti et al. [23] and Sagnia et al. [24] that produced the same results. However, the results obtained by Bwana et al. suggest that despite the sufficient comparability, there is still a risk of misdiagnosis resulting in lack of treatment [25]. An accuracy assessment of two different point-of-care testing methods, PIMA and Dymal, in comparison to BD FACSCount, was presented by Malagun et al. and revealed PIMA to be a highly accurate and accessible tool of POC analysis [26].
A comparative study including FACSCanto II and AQUIOS CL (Beckman Coulter) was described by Grossi et al., giving comparable results, although emphasising the need for some crucial adjustments, including the upgrade and enhancement of its function considering more advanced measurement [27]. Verbeek et al. compared both FACSCanto II and FACSLyric with the results of manual analysis of minimal residual disease. The results obtained suggest a similar strong correlation of both analysers’ results with the manual method [28].
The results we obtained are consistent with most of the comparative studies performed in other facilities since we also managed to find a strong correlation and good comparability of results between the two flow cytometry analysers we included in the study. However, some studies, especially those including the point-of-care testing procedures, indicate no influence of the staff training, experience and gating techniques on the accuracy of the results. We established that in our research the deviations shown in one of the comparative statistical methods, although the same sample was evaluated and the recommended controls remained positive, might derive from different gating techniques, as the analyses were carried out by different staff members. In conclusion, this study showed that only one statistical method that we used showed some disturbances; however, it should not influence the clinical outcome. It emphasises the importance of staff training and the normalisation of knowledge and techniques.